Data Structures & Algorithms in Swift Full Release Now Available!
The full release of our book Data Structures & Algorithms in Swift is now available — see what’s been added to the latest edition! By Manda Frederick.
Sign up/Sign in
With a free Kodeco account you can download source code, track your progress, bookmark, personalise your learner profile and more!
Create accountAlready a member of Kodeco? Sign in
Contents
Data Structures & Algorithms in Swift Full Release Now Available!
15 mins
Section IV: Sorting Algorithms
- Chapter 25: O(n²) Sorting Algorithms: O(n²) time complexity is not great performance, but the sorting algorithms in this category are easy to understand and useful in some scenarios. These algorithms are space efficient; they only require constant O(1) additional memory space. In this chapter, you’ll be looking at the bubble sort, selection sort, and insertion sort algorithms.us shapes and sizes.
- Chapter 27: Merge Sort: In this chapter, you’ll look at a completely different model of sorting. So far, you’ve been relying on comparisons to determine the sorting order. Radix sort is a non-comparative algorithm for sorting integers in linear time. There are multiple implementations of radix sort that focus on different problems. To keep things simple, in this chapter you’ll focus on sorting base 10 integers while investigating the least significant digit (LSD) variant of radix sort.
- Chapter 29: Radix Sort: A binary search tree facilitates fast lookup, addition, and removal operations. Each operation has an average time complexity of O(log n), which is considerably faster than linear data structures such as arrays and linked lists.
- Chapter 31: Heapsort: Heapsort is another comparison-based algorithm that sorts an array in ascending order using a heap. This chapter builds on the heap concepts presented in Chapter 21, “The Heap Data Structure.” Heapsort takes advantage of a heap being, by definition, a partially sorted binary tree.
- Chapter 33: Quicksort: Quicksort is another divide and conquer technique that introduces the concept of partitions and a pivot to implement high performance sorting. You‘ll see that while it is extremely fast for some datasets, for others it can be a bit slow.
Real-world examples help you apply the book’s concepts in a concrete and relevant way!
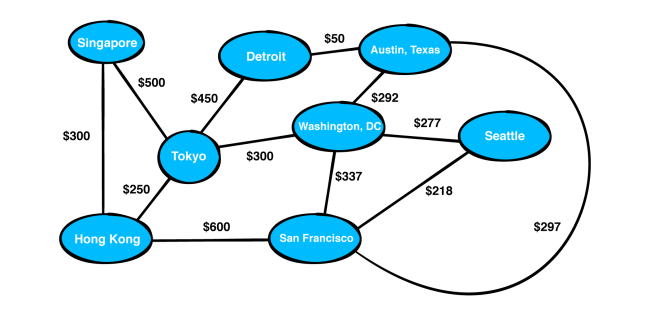
Section V: Graphs
- Chapter 35: Graphs: What do social networks have in common with booking cheap flights around the world? You can represent both of these real-world models as graphs! A graph is a data structure that captures relationships between objects. It is made up of vertices connected by edges. In a weighted graph, every edge has a weight associated with it that represents the cost of using this edge. This lets you choose the cheapest or shortest path between two vertices.
- Chapter 37: Breadth-First Search: In the previous chapter, you explored how graphs can be used to capture relationships between objects. Several algorithms exist to traverse or search through a graph’s vertices. One such algorithm is the breadth-first search algorithm, which can be used to solve a wide variety of problems, including generating a minimum spanning tree, finding potential paths between vertices, and finding the shortest path between two vertices.
- Chapter 39: Depth-First Search: In the previous chapter, you looked at breadth-first search where you had to explore every neighbor of a vertex before going to the next level. In this chapter, you will look at depth-first search, which has applications for topological sorting, detecting cycles, path finding in maze puzzles, and finding connected components in a sparse graph.
- Chapter 41: Dijkstra’s Algorithm: Have you ever used the Google or Apple Maps app to find the shortest or fastest from one place to another? Dijkstra’s algorithm is particularly useful in GPS networks to help find the shortest path between two places. Dijkstra’s algorithm is a greedy algorithm, which constructs a solution step-by-step, and picks the most optimal path at every step.
- Chapter 43: Prim’s Algorithm: In previous chapters, you’ve looked at depth-first and breadth-first search algorithms. These algorithms form spanning trees. In this chapter, you will look at Prim’s algorithm, a greedy algorithm used to construct a minimum spanning tree. A minimum spanning tree is a spanning tree with weighted edges where the total weight of all edges is minimized. You’ll learn how to implement a greedy algorithm to construct a solution step-by-step, and pick the most optimal path at every step.
The book moves beyond fundamentals to more advanced concepts, such as Dijkstra’s Algorithm!
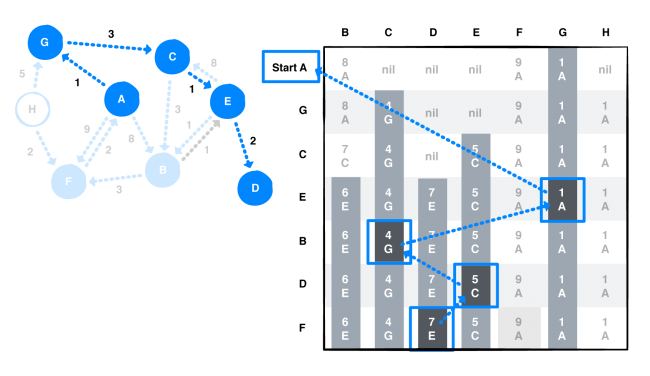
Data Structures and Algorithms in Swift will teach you how to implement the most popular and useful data structures, and when and why you should use one particular data structure or algorithm over another.
This set of basic data structures and algorithms will serve as an excellent foundation for building more complex and special-purpose constructs. And the high-level expressiveness of Swift makes it an ideal choice for learning these core concepts without sacrificing performance.
Get your own copy:
- If you’ve pre-ordered Data Structures & Algorithms in Swift, you can log in to the store and download the final version here.
- If you haven’t already bought this must-have addition to your development library, head over to our store page to grab your copy today!
Questions about the book? Ask them in the comments below!